AI-Integrated Processors and Chips: The Future of Intelligent Computing
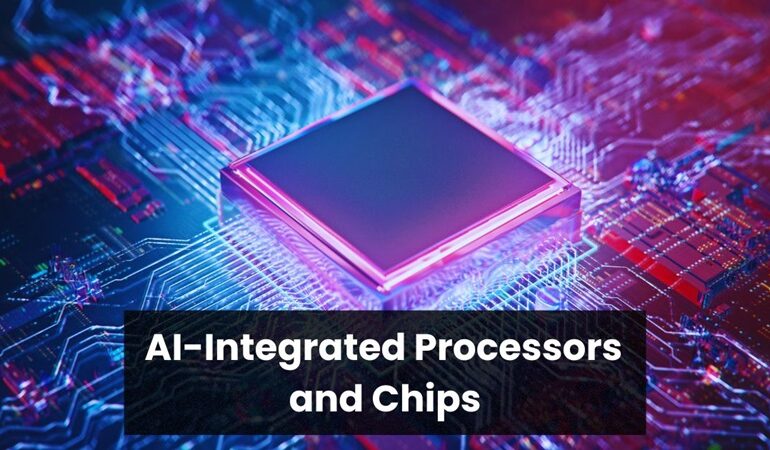
In the rapidly evolving world of technology, the integration of artificial intelligence (AI) into processors and chips is revolutionizing the way we think about computing power. No longer limited to raw computational capabilities, these AI-integrated processors are ushering in a new era of intelligent hardware, capable of performing complex tasks with unprecedented efficiency and accuracy.
The Evolution of Processors
Computing technology has undergone significant transformations over the past few decades, with processors evolving from simple CPUs to advanced GPUs. Central processing units (CPUs) were the standard for general-purpose computing tasks, but as the demand for high-performance computing grew, the need for more specialized processors became evident. This led to the development of graphics processing units (GPUs), which excel at parallel processing and have become essential for tasks like rendering graphics and running complex simulations.
The Role of AI in Computing
Artificial intelligence has become a key player in enhancing computational power. By integrating AI capabilities into processors, we can achieve greater efficiency and accuracy in various applications. AI algorithms, such as machine learning and deep learning, require significant computational resources, and AI-integrated processors are designed to meet these demands. These processors can handle complex tasks like data analysis, pattern recognition, and decision-making more effectively than traditional processors.
AI Accelerators: Revolutionizing Performance
One of the most significant developments in AI-integrated processors is the emergence of AI accelerators. These specialized chips are designed to accelerate the computationally intensive tasks associated with AI algorithms. By offloading these tasks from the central processing unit (CPU), AI accelerators can significantly boost performance and energy efficiency.
Overview of AI Accelerators
AI accelerators are specialized hardware components optimized for AI workloads. They provide the computational power needed for tasks like training machine learning models and executing inference tasks. These accelerators are typically used in data centers, cloud computing environments, and high-performance computing clusters.
Key Players in AI Accelerators
Companies like NVIDIA, Google, and Intel have been at the forefront of this revolution, developing powerful AI accelerators like the NVIDIA Tensor Core GPUs, Google’s Tensor Processing Units (TPUs), and Intel’s Nervana Neural Network Processors (NNPs). These accelerators are finding applications in a wide range of industries, from autonomous vehicles and robotics to healthcare and scientific research.
NVIDIA’s Tensor Core GPUs
NVIDIA has been a pioneer in the field of AI accelerators with its Tensor Core GPUs. These GPUs are designed to handle the intensive computational demands of AI algorithms, offering high performance and energy efficiency.
Features and Applications
NVIDIA’s Tensor Core GPUs are equipped with specialized cores that accelerate matrix operations, which are fundamental to AI workloads. They are used in various applications, including autonomous vehicles, robotics, and scientific research. The ability to perform complex calculations quickly and efficiently makes them ideal for tasks that require real-time processing and decision-making.
Google’s Tensor Processing Units (TPUs)
Google has developed its own line of AI accelerators known as Tensor Processing Units (TPUs). These custom-designed chips are optimized for Google’s machine learning framework, TensorFlow, and are used in Google’s data centers to power AI services.
Innovations and Benefits
Google’s TPUs are designed to handle the specific demands of machine learning workloads, providing high performance and efficiency. They are used in various Google products and services, including search, translation, and image recognition. By using TPUs, Google can deliver faster and more accurate AI-driven services to its users.
Intel’s Nervana Neural Network Processors (NNPs)
Intel has also made significant strides in the field of AI accelerators with its Nervana Neural Network Processors (NNPs). These processors are designed to accelerate deep learning workloads, offering high performance and efficiency.
Advancements and Use Cases
Intel’s NNPs are optimized for the computational demands of neural networks, providing the power needed for training and inference tasks. They are used in various applications, including autonomous vehicles, healthcare, and scientific research. The ability to handle large amounts of data quickly and efficiently makes them ideal for tasks that require real-time processing and decision-making.
Neuromorphic Computing: The Brain-like Processors
Another exciting development in AI-integrated processors is the field of neuromorphic computing. This approach aims to mimic the structure and function of the human brain, creating processors that can process information in a similar way to biological neural networks.
Definition and Potential
Neuromorphic computing involves designing processors that are inspired by the human brain’s architecture. These processors use specialized circuits that mimic the behavior of neurons and synapses, enabling them to perform complex tasks with high efficiency and low power consumption.
Intel’s Loihi Chip
Intel has been at the forefront of neuromorphic computing with its Loihi chip. This chip is designed to mimic the brain’s neural networks, providing high performance and efficiency for AI workloads.
Key Features and Applications
The Loihi chip is equipped with specialized circuits that enable it to perform complex tasks like pattern recognition and decision-making in real-time. It is used in various applications, including robotics, healthcare, and scientific research. The ability to process information in a brain-like manner makes it ideal for tasks that require high efficiency and low power consumption.
IBM’s TrueNorth
IBM has also made significant strides in the field of neuromorphic computing with its TrueNorth chip. This chip is designed to mimic the brain’s architecture, providing high performance and efficiency for AI workloads.
Innovations in Neuromorphic Computing
IBM’s TrueNorth chip is equipped with specialized circuits that enable it to perform complex tasks like pattern recognition and decision-making in real-time. It is used in various applications, including robotics, healthcare, and scientific research. The ability to process information in a brain-like manner makes it ideal for tasks that require high efficiency and low power consumption.
Samsung’s Neuromorphic Chips
Samsung has also made significant strides in the field of neuromorphic computing with its neuromorphic chips. These chips are designed to mimic the brain’s architecture, providing high performance and efficiency for AI workloads.
Advantages and Future Prospects
Samsung’s neuromorphic chips are equipped with specialized circuits that enable them to perform complex tasks like pattern recognition and decision-making in real-time. They are used in various applications, including robotics, healthcare, and scientific research. The ability to process information in a brain-like manner makes them ideal for tasks that require high efficiency and low power consumption.
AI in Mobile and Consumer Electronics
The integration of AI into processors is not limited to high-performance computing applications. Major chip manufacturers like Qualcomm, Apple, and Huawei have been incorporating AI capabilities into their mobile processors, enabling a range of intelligent features in smartphones and consumer electronics.
Qualcomm’s Snapdragon AI Processors
Qualcomm has been at the forefront of integrating AI capabilities into its Snapdragon processors. These processors are designed to handle the computational demands of AI workloads, providing high performance and efficiency.
Real-world Applications
Qualcomm’s Snapdragon AI processors are used in various applications, including real-time object recognition, augmented reality (AR) applications, and on-device natural language processing. These processors enhance the user experience by providing intelligent features and improving privacy and security by reducing the need to send data to the cloud for processing.
Apple’s A-Series Chips
Apple has also made significant strides in integrating AI capabilities into its A-series chips. These chips are designed to handle the computational demands of AI workloads, providing high performance and efficiency.
AI Capabilities and Features
Apple’s A-series chips are used in various applications, including real-time object recognition, augmented reality (AR) applications, and on-device natural language processing. These chips enhance the user experience by providing intelligent features and improving privacy and security by reducing the need to send data to the cloud for processing.
Huawei’s Kirin Chips
Huawei has also made significant strides in integrating AI capabilities into its Kirin chips. These chips are designed to handle the computational demands of AI workloads, providing high performance and efficiency.
AI Integration in Consumer Electronics
Huawei’s Kirin chips are used in various applications, including real-time object recognition, augmented reality (AR) applications, and on-device natural language processing. These chips enhance the user experience by providing intelligent features and improving privacy and security by reducing the need to send data to the cloud for processing.
AI for Autonomous Vehicles and Robotics
One of the most promising applications of AI-integrated processors is in the field of autonomous vehicles and robotics. These systems require real-time processing of vast amounts of sensor data, as well as complex decision-making and control algorithms.
NVIDIA’s Drive Platform
NVIDIA has been at the forefront of developing AI processors for autonomous vehicles with its Drive platform. This platform is designed to handle the computational demands of autonomous driving, providing high performance and efficiency.
Features and Advancements
NVIDIA’s Drive platform is equipped with specialized processors that enable it to process data from cameras, radar, and lidar sensors in real-time. This platform is essential for enabling advanced driver assistance systems (ADAS) and fully autonomous driving capabilities.
Intel’s Mobileye
Intel has also made significant strides in developing AI processors for autonomous vehicles with its Mobileye platform. This platform is designed to handle the computational demands of autonomous driving, providing high performance and efficiency.
Role in Autonomous Driving
Intel’s Mobileye platform is equipped with specialized processors that enable it to process data from cameras, radar, and lidar sensors in real-time. This platform is essential for enabling advanced driver assistance systems (ADAS) and fully autonomous driving capabilities.
Challenges in AI Integration
While the integration of AI into processors and chips offers numerous benefits, it also presents several challenges. One of the primary concerns is the energy consumption and heat dissipation of these powerful AI-integrated processors, which can be a significant issue in mobile and embedded devices.
Addressing Privacy and Security
Privacy and security are critical considerations in the integration of AI into processors. AI-integrated processors may handle sensitive data and make decisions that could have significant consequences. Ensuring that these processors are secure and that privacy is maintained is essential for their widespread adoption.
Ethical Considerations
The development and use of AI-integrated processors also raise ethical considerations. It is important to ensure that these processors are used responsibly and that their decisions are transparent and fair. Addressing these ethical concerns is essential for gaining public trust and ensuring the responsible use of AI technology.
The Future of AI-Integrated Processors
Despite the challenges, the integration of AI into processors and chips is poised to shape the future of intelligent computing. As AI algorithms and hardware continue to evolve, we can expect to see even more powerful and efficient AI-integrated processors, capable of tackling increasingly complex tasks.
Advancements in AI Algorithms
Advancements in AI algorithms are driving the development of more powerful and efficient AI-integrated processors. These advancements are enabling new applications and improving the performance of existing ones. As AI algorithms continue to evolve, we can expect to see even greater capabilities from AI-integrated processors.
Collaboration Between Hardware and Software
The integration of AI into processors requires close collaboration between hardware and software developers. By working together, these developers can create optimized solutions that take full advantage of AI capabilities. This collaboration is essential for achieving the best performance and efficiency from AI-integrated processors.
AI in Healthcare
The integration of AI into processors is transforming the field of healthcare. AI-integrated processors are being used to analyze medical data, diagnose diseases, and develop personalized treatment plans. These processors are enabling new levels of accuracy and efficiency in medical diagnostics and treatment.
AI in Scientific Research
AI-integrated processors are also transforming the field of scientific research. These processors are being used to analyze large datasets, run complex simulations, and make new discoveries. By providing the computational power needed for advanced research, AI-integrated processors are enabling groundbreaking discoveries in fields like physics, biology, and chemistry.
AI in Everyday Life
The integration of AI into processors is also enhancing everyday life. AI-integrated processors are being used in smart home devices, personal assistants, and other consumer electronics. These processors provide intelligent features that make everyday tasks easier and more convenient.
Case Studies of AI Integration
There are numerous success stories of AI integration across various industries. From autonomous vehicles to healthcare, AI-integrated processors are enabling new levels of innovation and efficiency. These case studies demonstrate the potential of AI technology and its impact on different fields.
The integration of AI into processors and chips is revolutionizing the world of intelligent computing. From AI accelerators and neuromorphic computing to mobile processors and autonomous vehicles, AI-integrated processors are enabling new levels of innovation and efficiency. Despite the challenges, the future of AI-integrated processors is bright, with advancements in AI technology driving new applications and transforming various industries. As we continue to push the boundaries of what is possible with intelligent hardware, we can look forward to a future where computing power and artificial intelligence are seamlessly integrated, enabling new levels of innovation and technological advancement.
FAQs
1. What are AI-integrated processors?
- AI-integrated processors are specialized chips designed to handle the computational demands of AI workloads. They provide high performance and efficiency for tasks like machine learning and deep learning.
2. How do AI accelerators work?
- AI accelerators are specialized hardware components optimized for AI workloads. They offload computationally intensive tasks from the CPU, boosting performance and energy efficiency.
3. What is neuromorphic computing?
- Neuromorphic computing involves designing processors that mimic the structure and function of the human brain. These processors use specialized circuits to perform complex tasks with high efficiency and low power consumption.
4. How are AI-integrated processors used in autonomous vehicles?
- AI-integrated processors are used in autonomous vehicles to process data from sensors in real-time. They enable advanced driver assistance systems (ADAS) and fully autonomous driving capabilities.
5. What are the challenges of integrating AI into processors?
- The primary challenges of integrating AI into processors include energy consumption, heat dissipation, privacy, security, and ethical considerations.